The Royal Bolton Hospital is among a growing number of health-care facilities around the world that are turning to AI to help manage the coronavirus pandemic. Many are using such technologies for the first time under the pressure of staff shortages and overwhelming patient loads. In parallel, dozens of AI firms have developed new software or revamped existing tools, hoping to cash in by cultivating new client relationships that will continue after the crisis is over.
The pandemic, in other words, has turned into a gateway for AI adoption in health care—bringing both opportunity and risk. On the one hand, it is pushing doctors and hospitals to fast-track promising new technologies. On the other, this accelerated process could allow unvetted tools to bypass regulatory processes, putting patients in harm’s way.
“At a high level, artificial intelligence in health care is very exciting,” says Chris Longhurst, the chief information officer at UC San Diego Health. “But health care is one of those industries where there are a lot of factors that come into play. A change in the system can have potentially fatal unintended consequences.”
“It’s really an attractive solution”
Before the pandemic, health-care AI was already a booming area of research. Deep learning, in particular, has demonstrated impressive results for analyzing medical images to identify diseases like breast and lung cancer or glaucoma at least as accurately as human specialists. Studies have also shown the potential of using computer vision to monitor elderly people in their homes and patients in intensive care units.
But there have been significant obstacles to translating that research into real-world applications. Privacy concerns make it challenging to collect enough data for training algorithms; issues related to bias and generalizability make regulators cautious to grant approvals. Even for applications that do get certified, hospitals rightly have their own intensive vetting procedures and established protocols. “Physicians, like everybody else—we’re all creatures of habit,” says Albert Hsiao, a radiologist at UCSD Health who is now trialing his own covid detection algorithm based on chest x-rays. “We don’t change unless we’re forced to change.”
As a result, AI has been slow to gain a foothold. “It feels like there’s something there; there are a lot of papers that show a lot of promise,” said Andrew Ng, a leading AI practitioner, in a recent webinar on its applications in medicine. But “it’s not yet as widely deployed as we wish.”
QURE.AI
Pierre Durand, a physician and radiologist based in France, experienced the same difficulty when he cofounded the teleradiology firm Vizyon in 2018. The company operates as a middleman: it licenses software from firms like Qure.ai and a Seoul-based startup called Lunit and offers the package of options to hospitals. Before the pandemic, however, it struggled to gain traction. “Customers were interested in the artificial-intelligence application for imaging,” Durand says, “but they could not find the right place for it in their clinical setup.”
The onset of covid-19 changed that. In France, as caseloads began to overwhelm the health-care system and the government failed to ramp up testing capacity, triaging patients via chest x-ray—though less accurate than a PCR diagnostic—became a fallback solution. Even for patients who could get genetic tests, results could take at least 12 hours and sometimes days to return—too long for a doctor to wait before deciding whether to isolate someone. By comparison, Vizyon’s system using Lunit’s software, for example, takes only 10 minutes to scan a patient and calculate a probability of infection. (Lunit says its own preliminary study found that the tool was comparable to a human radiologist in its risk analysis, but this research has not been published.) “When there are a lot of patients coming,” Durand says, “it’s really an attractive solution.”
Vizyon has since signed partnerships with two of the largest hospitals in the country and says it is in talks with hospitals in the Middle East and Africa. Qure.ai, meanwhile, has now expanded to Italy, the US, and Mexico on top of existing clients. Lunit is also now working with four new hospitals each in France, Italy, Mexico, and Portugal.
In addition to the speed of evaluation, Durand identifies something else that may have encouraged hospitals to adopt AI during the pandemic: they are thinking about how to prepare for the inevitable staff shortages that will arise after the crisis. Traumatic events like a pandemic are often followed by an exodus of doctors and nurses. “Some doctors may want to change their way of life,” he says. “What’s coming, we don’t know.”
“Once people start using our algorithms, they never stop”
Hospitals’ new openness to AI tools hasn’t gone unnoticed. Many companies have begun offering their products for a free trial period, hoping it will lead to a longer contract.
“It’s a good way for us to demonstrate the utility of AI,” says Brandon Suh, the CEO of Lunit. Prashant Warier, the CEO and cofounder of Qure.ai, echoes that sentiment. “In my experience outside of covid, once people start using our algorithms, they never stop,” he says.
Both Qure.ai’s and Lunit’s lung screening products were certified by the European Union’s health and safety agency before the crisis. In adapting the tools to covid, the companies repurposed the same functionalities that had already been approved.
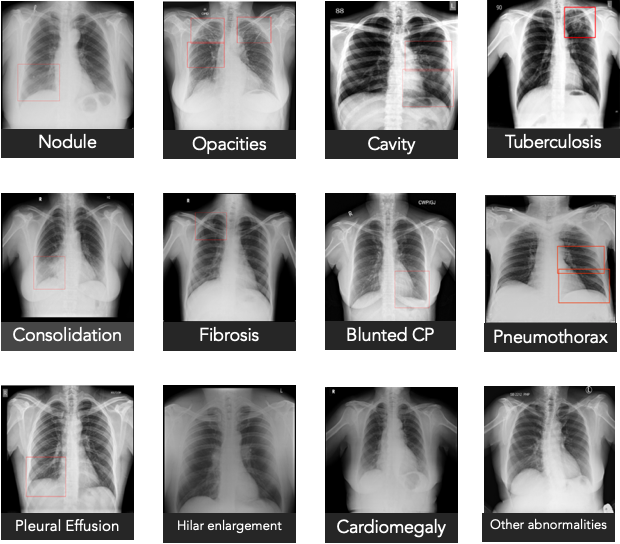
QURE.AI
Qure.ai’s qXR, for example, uses a combination of deep-learning models to detect common types of lung abnormalities. To retool it, the firm worked with a panel of experts to review the latest medical literature and determine the typical features of covid-induced pneumonia, such as opaque patches in the image that have a “ground glass” pattern and dense regions on the sides of the lungs. It then encoded that knowledge into qXR, allowing the tool to calculate the risk of infection from the number of telltale characteristics present in a scan. A preliminary validation study the firm ran on over 11,000 patient images found that the tool was able to distinguish between covid and non-covid patients with 95% accuracy.
But not all firms have been as rigorous. In the early days of the crisis, Malik exchanged emails with 36 companies and spoke with 24, all pitching him AI-based covid screening tools. “Most of them were utter junk,” he says. “They were trying to capitalize on the panic and anxiety.” The trend makes him worry: hospitals in the thick of the crisis may not have time to perform due diligence. “When you’re drowning so much,” he says, “a thirsty man will reach out for any source of water.”
Kay Firth-Butterfield, the head of AI and machine learning at the World Economic Forum, urges hospitals not to weaken their regulatory protocols or formalize long-term contracts without proper validation. “Using AI to help with this pandemic is obviously a great thing to be doing,” she says. “But the problems that come with AI don’t go away just because there is a pandemic.”
UCSD’s Longhurst also encourages hospitals to use this opportunity to partner with firms on clinical trials. “We need to have clear, hard evidence before we declare this as the standard of care,” he says. Anything less would be “a disservice to patients.”
To have more stories like this delivered directly to your inbox, sign up for our Webby-nominated AI newsletter The Algorithm. It’s free.